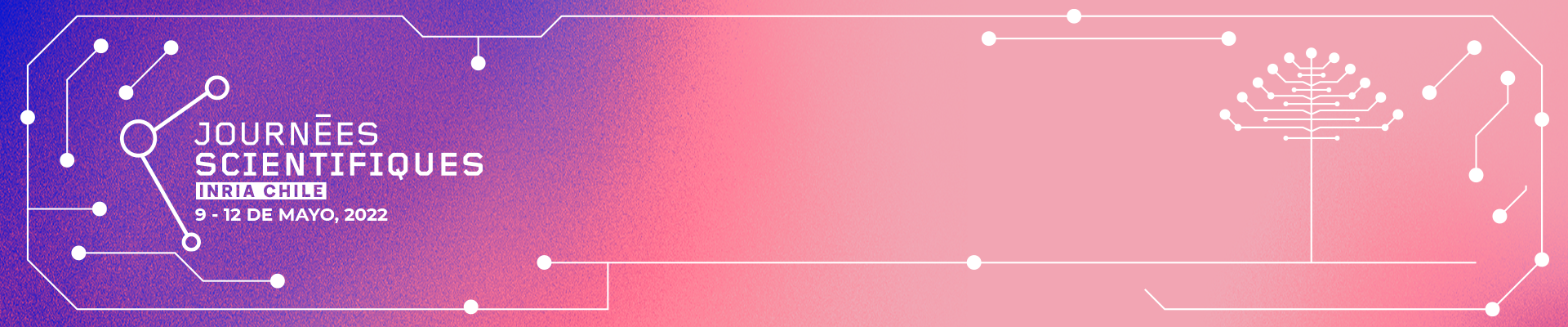
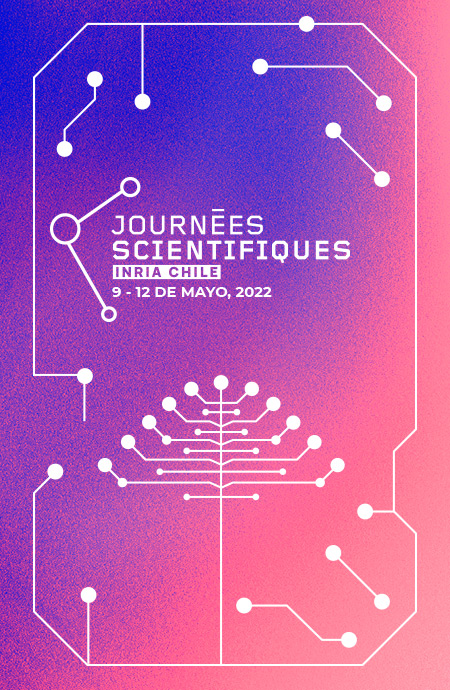
Marc Schoenauer is a senior researcher at Inria for more than 20 years. Previously, he was at the Centre National de la Recherche Scientifique (CNRS) and at the Centre de Mathématiques Appliquées (CMAP) of the École Polytechnique. He founded the TAO team at Inria Saclay with Michèle Sebag in 2003. His work is on the frontier between Evolutionary Computing and Machine Learning; and he has published more than 150 articles and (co)advisored 35 PhD students. He has been president of ACM-SIGEVO (2015-2019) and president of AFIA (2002-2004) and supported Cédric Villani in drafting his report on the French Strategy for AI presented in March 2018.
The emergence of Deep Learning has caused incredible successes in the field of Artificial Intelligence, but now it seems to be reaching a glass ceiling in terms of reliability in its favorite domains, such as images and videos, NLP, and games, among others. This happens, while simultaneously it is expanding horizontally into all scientific domains. In this keynote, I will argue that in both contexts, hybridization of deep learning and other fields is the key to future beneficial advances, and we will illustrate these arguments with examples.
Indeed, there is currently no way to ensure that a Deep Neural Network (DNN) will behave as trained, and there are numerous examples of unwanted, catastrophic behaviors because of adversarial attacks or contexts that are too different. We will probably never be able to reach a 100% reliability –or even a 99.99% –, but there are many application domains that are not critical, in which a certain error range is admissible; or where the AI systems only does some pre-screening of clear cases, but asks the human expert in case of doubt. Such man-machine collaboration is one of the potentialities, if we take care of keeping human control over the final decision.
This is hard to tell. Some (famous) scientists think DL is everything, and it will be able to replace all sciences –which is cartoonish, but not totally exaggerated. Some other scientists have serious doubts about that, discussing that we have already reached the top of the hill, thus DL is already collapsing. Technically, the limits of DL are hard to identify. I think we are very far, for instance, of an Artificial General Intelligence (AGI), but this does not mean that great progress cannot be achieved on the way to AGI, even if it will never be even close to be reached.
However, the main hurdles that DL faces are not just technical, they ought to deal with trustworthiness –that includes explainability, robustness/certification, fairness, privacy, computational/energy cost, lack of common sense, among many other issues.
Well, that is the title of my keynote. Yes, I believe that is true. Even more so for public research facing big tech companies, like GAFAMs or BATXs, because AI is becoming a mandatory tool in every scientist toolbox, and great progress is expected in other domains thanks to AI, but also in AI because of the new problems other domains will face. And public research covers all possible areas, something big techs cannot compete with, so there still are niches where public research can cover and accomplish before big techs can.
When you believe that hybridization is the future of AI, you also believe that collaboration (across both, geographical and disciplinary borders) is the only way to go. And most players today in AI play the game of reproducibility, transparency and openness. At least those who publish in well-known conferences. Because, of course, we do not know of the others…
First, you need the politicians, who decide where money for research should go, to be convinced; and I think the Villani report is a consequence, not a cause of such conviction of the French politicians in power at the time. But then, one realizes that your degrees of freedom are rather limited. Some other initiatives are already going, and basically you can only influence at the margin. But you get to meet very diverse and interesting people, and this is always fascinating.
As for Latin America, I confess I do not have enough context to be able to say anything intelligent. One thing comes to my mind, though: in France, when the French AI Strategy was ordered, we had just undergone a change of politicians –the arrival of President Macron– and this may explain this will to change things. So maybe, is now the right time for Chile? You need someone who knows the scientific and political landscape very well, to lead the game.